Introduction
Artificial Intelligence (AI) is ushering in a new era across different sectors, including healthcare. The incorporation of AI into medical research is expected to induce substantial transformations, augmenting the precision and effectiveness of diagnosis, treatment, and patient care.
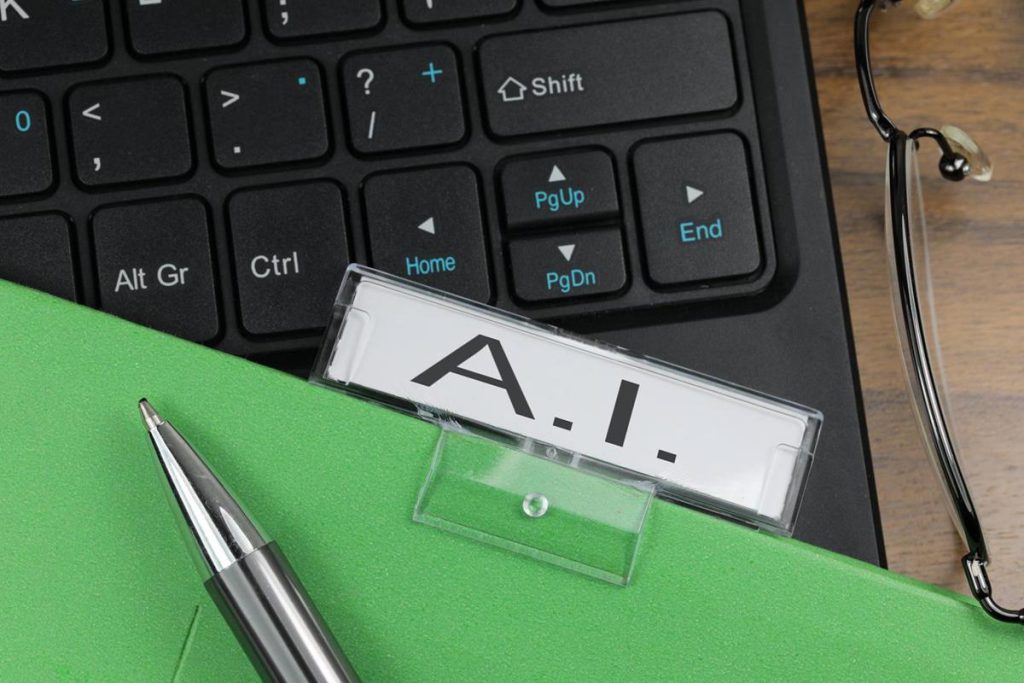
AI in Disease Diagnosis and Treatment
One of the most exciting applications of AI in medical research lies in its potential for disease diagnosis and treatment. Machine learning algorithms have the ability to process and analyze vast amounts of data from medical records, genetic databases, and medical literature. This enables them to detect patterns that humans may overlook. These patterns can help in forecasting the course of diseases and proposing personalized treatment strategies.
Here are some ways AI is being used in Disease Diagnosis and Treatment:
Pattern Recognition:
AI has the ability to process enormous quantities of data from medical records, genetic databases, and medical publications to uncover patterns that might miss human detection. These patterns can assist in forecasting how a disease will progress and in devising personalized treatment strategies.
Medical Imaging:
AI can aid in the interpretation of medical images such as ultrasound, magnetic resonance imaging (MRI), mammography, genomics, and computed tomography (CT) scans. This enhances the precision and shortens the time required for diagnosis.
Self-Diagnosis
AI chatbots like OpenAI’s ChatGPT, the latest version of Microsoft’s search engine Bing (built on OpenAI’s software), and Google’s Med-PaLM are being utilized for self-diagnosis. These AI systems offer accuracy that surpasses a simple Google search.
Assisting Physicians:
AI is designed not to replace physicians but to bolster their decisions and provide suggestions based on the analysis of vast healthcare data. This data can include electronic health records (EHRs), symptom data, medical images, doctor reports, and more.
Drug Discovery and Development
AI has the potential to accelerate the process of drug discovery. Traditionally, the journey of a new drug from discovery to market can span up to 15 years and cost billions of dollars. However, AI can analyze chemical compounds and predict their impacts on the human body, significantly cutting down the time and cost associated with drug discovery.
AI is revolutionizing the domain of drug discovery and development in several notable ways:
Access to New Biology
AI can add value to small-molecule drug discovery by uncovering new biological insights. This technology can tackle numerous challenges and limitations inherent in traditional R&D.
Improved Chemistry
AI can potentially enhance existing chemistry or even develop new chemical processes. This could pave the way for the discovery of novel drugs.
Enhanced Success Rates:
AI can potentially boost drug discovery success rates by analyzing data and making precise predictions.
Accelerated and Cost-Effective Discovery Processes:
AI has the potential to expedite and reduce the cost of discovery processes. This is particularly significant considering that traditionally, the journey from discovering a new drug to bringing it to market can span up to 15 years and cost billions of dollars.
Comprehensive Drug Discovery Capabilities:
Certain AI-centric drug discovery firms have established comprehensive drug discovery capabilities and internal pipelines. This has given rise to a new generation of biotech companies.
Predictive Analytics in Healthcare
Predictive analytics is a domain where AI can have a substantial influence. Predictive analytics in healthcare is an advanced analytics field that leverages both contemporary and historical data to predict activities, behaviors, and trends. It employs statistical and modeling techniques to predict future outcomes based on present and past data.
Here’s how AI is amplifying the capabilities of predictive analytics in healthcare:
Early Identification:
Predictive analytics can assist in identifying early indicators of patient deterioration in the ICU and general ward. This can be especially beneficial in the ICU, where timely intervention can be critical when a patient’s condition is on the verge of worsening.
Preventing Hospital Readmissions:
AI can pinpoint patients at risk in their homes to prevent hospital readmissions. This can be instrumental in not just curing but also preventing health issues.
Predicting Diseases/Disorders:
AI is used in predictive analytics to examine patient datasets and estimate the probability of certain diseases/disorders. Recent research has demonstrated that AI can detect conditions that are traditionally challenging to detect or diagnose, including rare genetic and neurodegenerative diseases.
Integrating External Factors:
As AI advances, external elements such as social determinants and lifestyle choices may be integrated into these models. It’s estimated that around 40% to 50% of an individual’s health outcomes are determined by their behavior and 20% by their physical and social environment, while genetics contribute to only about 30%.
Boosting Cybersecurity:
The healthcare industry can block high-risk activities, oversee their data in real-time, and apply multi-factor authentication (MFA) to promote cybersecurity by employing AI-based predictive analytics solutions. This can aid in averting data breaches, safeguarding patient information, and ensuring uninterrupted care.
Clinical Trial Research:
AI can assist in identifying appropriate participants for clinical trials based on a broad set of criteria, thereby enhancing the effectiveness of clinical research.
Designing Clinical Trials:
AI can be utilized to simulate the structure of a study and identify potential challenges early on, using a combination of new and existing data from prior trials. This minimizes the likelihood of failure and ensures that studies are as streamlined as possible, benefiting patients and pharmaceutical companies.
Patient Recruitment and Retention:
AI can aid in the process of finding potential participants for a study and predicting the likelihood of their withdrawal, thereby enhancing patient recruitment and retention.
Analyzing Chemical Compounds:
AI is increasingly used in preclinical and clinical trials to analyze chemical compounds. Sophisticated algorithms can swiftly sift through extensive chemical compound databases and pinpoint those with the highest likelihood of binding to a target. This accelerates the exploration of the chemical realm for drug developers.
Promoting Clinical Cycle Times:
Artificial intelligence has the potential to expedite clinical trial processes, thereby enhancing productivity and the results of clinical development, all while reducing costs.
Enhancing Clinical Research with AI:
As healthcare continues to evolve towards a data-centric and personalized medicine model, both companies and regulatory bodies must adopt bespoke AI solutions. These solutions can facilitate faster and more efficient clinical research.
Final words
AI holds the promise of transforming medical research through enhanced disease diagnosis, expedited drug discovery, and the enablement of predictive analytics. Yet, it’s essential to navigate the accompanying ethical issues carefully. As we further incorporate AI into healthcare, our aim should be to strike a balance between fostering innovation and upholding ethics.