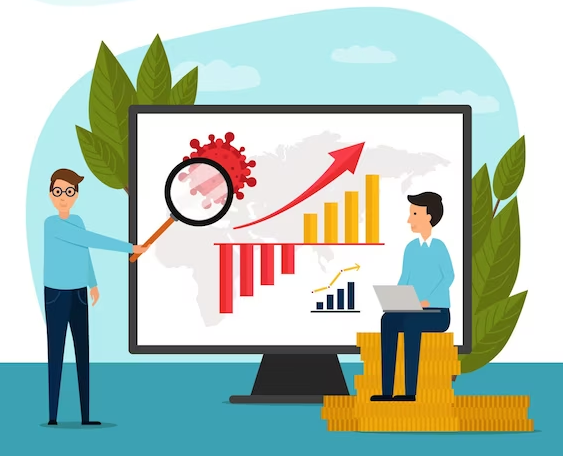
survival analysis is a branch of statistical methods that analysis time-to-event data. More precisely, time-to-event data are those that interest outcome is the time until a specific event occurs. These outcomes are varied from one field to another. For example, in public health, they could be disease occurrence, death, heart attacks, recovery, etc. Historically, this branch was developed firstly in biomedical science to depict the onset of specific ills. Still, today, its domain has expanded in various fields like engineering, insurance, and so on.
Time-to-event means this study branch collects and analyzes data on time until death (or other determinant outcomes) happens. It includes different data analysis methods, including life table analysis, time-to-failure methods, and time-to-death analysis. Based on the concept of such analysis, this kind of study is obviously time-consuming, and several factors may affect it. So, this article aims to explain why different researchers do this analysis, why it is essential in different aspects of science, and what are the methods, and common functions used in such analysis.
Why do researchers and clinicians use survival analysis?
Survival analysis is highly important when the time between exposure and a specific event is vital from clinical aspects. For example, consider a study that assesses the mortality of patients with different tumor sizes (˃1 cm V.s ˃5 cm) in a 5-year period. The hypothesis was that the survival rate in the former group was 85%, while it was 52% in the latter. In addition, such research may consider year-by-year survival and showed that the 2-year survival rate for the latter group was about 70%. These statistics help researchers and clinicians to calculate the golden time for treatment. They may also use such data to introduce a new treatment to increase the life expectancy of patients.
Characteristics and fundamental goals of Survival Data in health-related analysis
Survival data have several characteristics that should be completely defined. These are as follows:
- Appropriately define the time since entry into the study
- Appropriately define the time that the study ends. This means the event or failure, i.e., death, heart attack, recovery from surgery, etc., occurs.
- Each enrolled patient should be followed from the baseline date; for example, if the study regards cancer, the baseline date would be the diagnosis date or surgery date, and he/she followed until death or the end of the study.
- The survival data are essentially positive since these are collected during a time series, and time is a positive value.
Survival analysis also has primary goals, including:
- Use survival data, e.g., time to MI for patients who suffer from chronic blood pressure to evaluate and interpret survival and hazards functions
- Compare survival or hazard function: for example, for data on heart disease patients with chronic high blood pressure treated with conventional and new drugs in a randomized controlled trial.
- Investigate the relationship between variables (such as patient weight, cholesterol level, smoking habit, etc.) that affects survival time in people suffering from acute heart disease.
Special features used in Survival analysis
To do a perfect survival analysis, you need specific methods and techniques since these are time series data, making them different from other types of data. Specific features would be as follow:
Censoring
This happens when some enrolled people can’t meet the event deadline. For instance, censoring happens when a person drops out of a study before it ends or when the study ends before the ultimate event arrives for some enrolls. There are three types of censoring: right-censored, i.e., the event occurs after the observation period; left-censored means that the event happens before the observation period; and interval-censored, which means that the event occurs during time intervals.
Non-normality
Due to the nature of such data, this analysis usually suffers from non-normality distribution. These data may be skewed or heavy-tailed distributions due to different reasons. You cannot leverage statistical analysis methods that assume normality for such data. Examples of statistical analysis that assume normality is linear regression, different compare means methods like Duncan, and different t-test analyses.
Time-dependent covariates
These are variables that may change over time and influence survival time. For example, for patients with heart disease, variables like blood pressure, body weight, smoking habits, etc., may change over time, affecting death or heart attack risks. In survival analysis, you have to consider these variables and define how they can affect the survival or hazard function.
Different functions used in Survival analysis
In survival analysis, researchers use some common functions. Some of the most conventional are presented below:
Survival function
This function, called survivor or reliability function, provides the probability of surviving past time t. researchers generally estimate it using the Kaplan-Meier method or parametric models.
Hazard function
This function called the failure rate or force of mortality, provides the instantaneous failure rate at time t, divided into surviving up to time t. this function is generally evaluated by Cox proportional hazards or parametric models.
Cumulative hazard function
This function (Ht) provides the total accumulated risk of failure up to time t. Cumulative hazard function relates to survival function as follows:
Long-rank test
It is classified as a non-parametric test and helps researchers to compare survival curves between two groups or more. Using this test, a researcher can find if there are any significant differences in the survival distributions between groups or not.
Cox proportional hazards model
It is belonged to hazards models and is considered a semi-parametric model. It relates the function to a group of variables specifying a parametric form for the baseline of the hazard function. This model assumes that the variables mentioned above have multiplier effects on hazard function, and these effects are constant during the time (i.e. proportional hazards assumption).
Parametric survival models
These are models that determine a parametric baseline for the hazard function and the covariate’s effect on the hazard function.
Statistical techniques used in survival analysis in public health research
The assumption of parametric statistics is based on the distribution of the population of the sample is come from. On the contrary, non-parametric statistics are not based on such an assumption, so the sample data can be achieved from the population without any specific distribution. Usually, non-parametric models are used in survival analysis, but some parametric models can also be used. The most common parametric and non-parametric models are as follows:
Non-parametric methods
- Kaplan-Meier method: to estimate the survival function
- Cox proportional hazards model: for identifying risk factors and assessing adjusted risk ratios.
Parametric methods
- Accelerated failure time model
- Hazard functions: for the exponential, Weibull, gamma, Gompertz, lognormal, and log-logistic distributions.
conclusion
survival analysis is a valuable tool designed to analyze time-to-event data, specifically in biomedical science. This statistical method requires unique statistical methods, including censoring, non-normality of data, time-dependent covariants, etc.